Relationship Commerce
Turning data into dollars
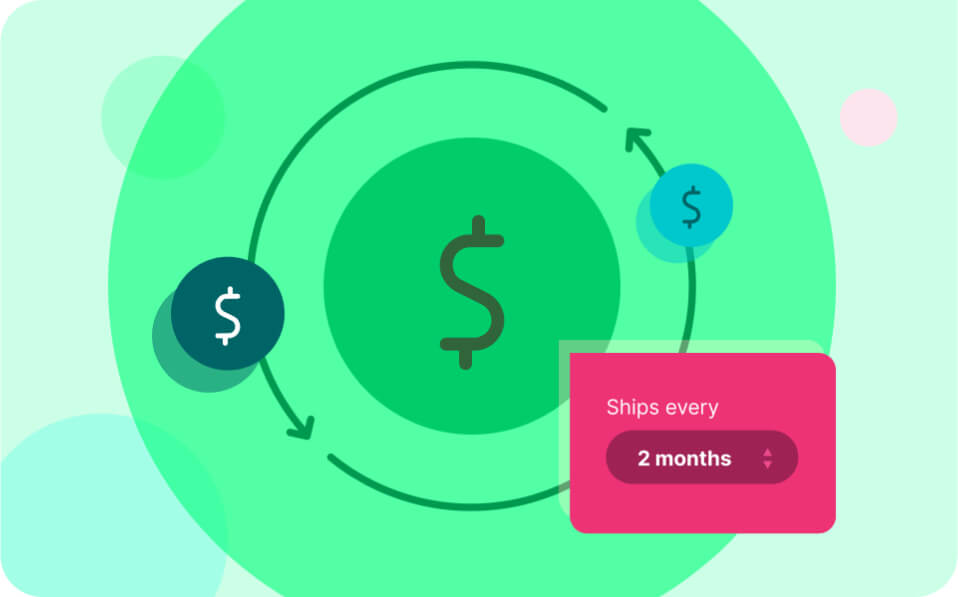
3 key ways data science powers Relationship Commerce.
Mention the term “data science” at a party and people’s eyes will glaze over; mention it an an investor meeting and suitcases of cash will materialize. Mention it anywhere else and people will congratulate you on combining two buzzy words into an even buzzier phrase, but will have very little idea of what you’re talking about.
And yet, despite the fact that it’s largely misunderstood, data science is key to making Relationship Commerce work. Without the tools to analyze data correctly, it would be impossible for any retailer to predict customer behavior — and impossible to use those predictions to create a better experience for both retailers and consumers.
Traditionally, data has been used by e-commerce retailers to convert the browser into a buyer, through an understanding of consumers’ browsing histories and demographic data.
Relationship Commerce, however, harnesses data to predict the timing and composition of the customer’s next order, thereby removing friction from shopping through a precise understanding of the how, when, where, and what of each individuals’ preferences.
Both models might use the very same data sets, but they take a radically different approach when it comes to parsing, analyzing, and understanding those numbers.
So how exactly is data science used in a Relationship Commerce setting? There are three major ways that it differs from the traditional way of doing things.
1. IT’S ABOUT CUSTOMER RETENTION, NOT CUSTOMER ACQUISITION.
Through a suite of data analytics tools, e-commerce sites have become effective in determining purchase intent in individual shopping, based on the signals from a consumer’s solitary session along with product affinities (which products go well with others) and other “static” techniques.
But the goals of RC are different, focusing instead on one specific piece of the retail puzzle: recurring customers, who will drive the most value by returning and shopping multiple times. Engaging those individuals comes down to two things: 1. predicting a future moment of need to offer accurate and convenient re-order prompts, and, 2. fine-tuning offerings, operations, and experience to better meet customers’ evolving needs.
The challenge of data science in this setting is to distill and isolate a consumer’s ideal purchase habits. That’s not simply a matter of gathering and parsing data sets, but also making inferences based on noisy or inconsistent data, distortion of incentives on purchase behavior, or incomplete information from third party providers.
The challenge of data science is to distill & isolate a consumer’s ideal purchase habits, while also making inferences based on incomplete purchase data.
2. IT’S ABOUT THE INDIVIDUAL, NOT THE DEMOGRAPHIC.
Every consumer’s habits are unique, and the problem RC-centered data scientists face when trying to understand them is learning their shopping habits.
It’s a fundamental shift in how retailers engage with their consumers, thus the data that’s traditionally been used by commercial interests — demographics, income, time of day, etc. — aren’t the crucial data points.
People’s consistency in purchasing varies widely in different product categories. Patterns of buying sneakers, for example, are relatively stable (though still unique, and subject to many of the issues detailed above). But it’s not as easy to predict when consumers will purchase a new video game.
But when data science is successful in understanding an individual’s habits and cadences, Relationship Commerce is better able to engage consumers through re-order reminders and special offers. That also means the offerings from retailers are customized for specific consumers, whether it be re-order alerts or subscription box programs.
3. IT’S ABOUT HELPING CONSUMERS MEEET THEIR GOALS, NOT MAX THEIR CREDIT CARDS.
One central thesis of Relationship Commerce is that customers are not making most of their purchases on a whim — they’re doing it to address concrete needs. So data needs to be able to answer the question, “What is a consumer trying to accomplish by making this purchase?”
The challenge for data scientists in this setting is to understand a consumer’s overall goals over a long period of time and make as much sense of that as possible. Suddenly consumers aren’t faced with the all-too-familiar prospect of running out of dishwasher soap or toilet paper.
When data science is successful in understanding an individual’s habits and cadences, the offerings from retailers are customized for specific consumers.
Traditional recommendations have tended to generate a flattened view of a customer – static, without a serious understanding of time – which leads to recommendations that look extremely similar to whatever they just bought. Which is very useful (and often effective) – but stops short of the level of usefulness that Relationship Commerce aspires to.
Relationship Commerce recommendations build a narrative to understand exactly when to recommend different consumables and so create an experience that is far more relevant and dynamic. Like traditional recommendation systems, RC takes into account the ‘what’ of customer needs and preferences, but also how the what depends on the when – how multiple needs are interwoven over time.
Which, to delve one layer deeper into the semantics, means that data can be used to get close to understanding why a consumer is purchasing certain products — Creatine and Protein Powder, for example. Thus it’s possible to infer an individual’s goal (more than likely building muscle mass, in this case) and recommend complementary products that other customers have purchased, or even a comparable product when something is out of stock. And that kind of experience — where the retailers seems to intuitively “get” what the customer wants, before they want it — is why Relationship Commerce represents the future of retail.